Regarding the Quincunx or Galton Board
12/27/18
Over at Bayesian Spectacles they wrote A Galton Board Demonstration of Why All Statistical Models are Misspecified. In it they discuss the quincunx or Galton board
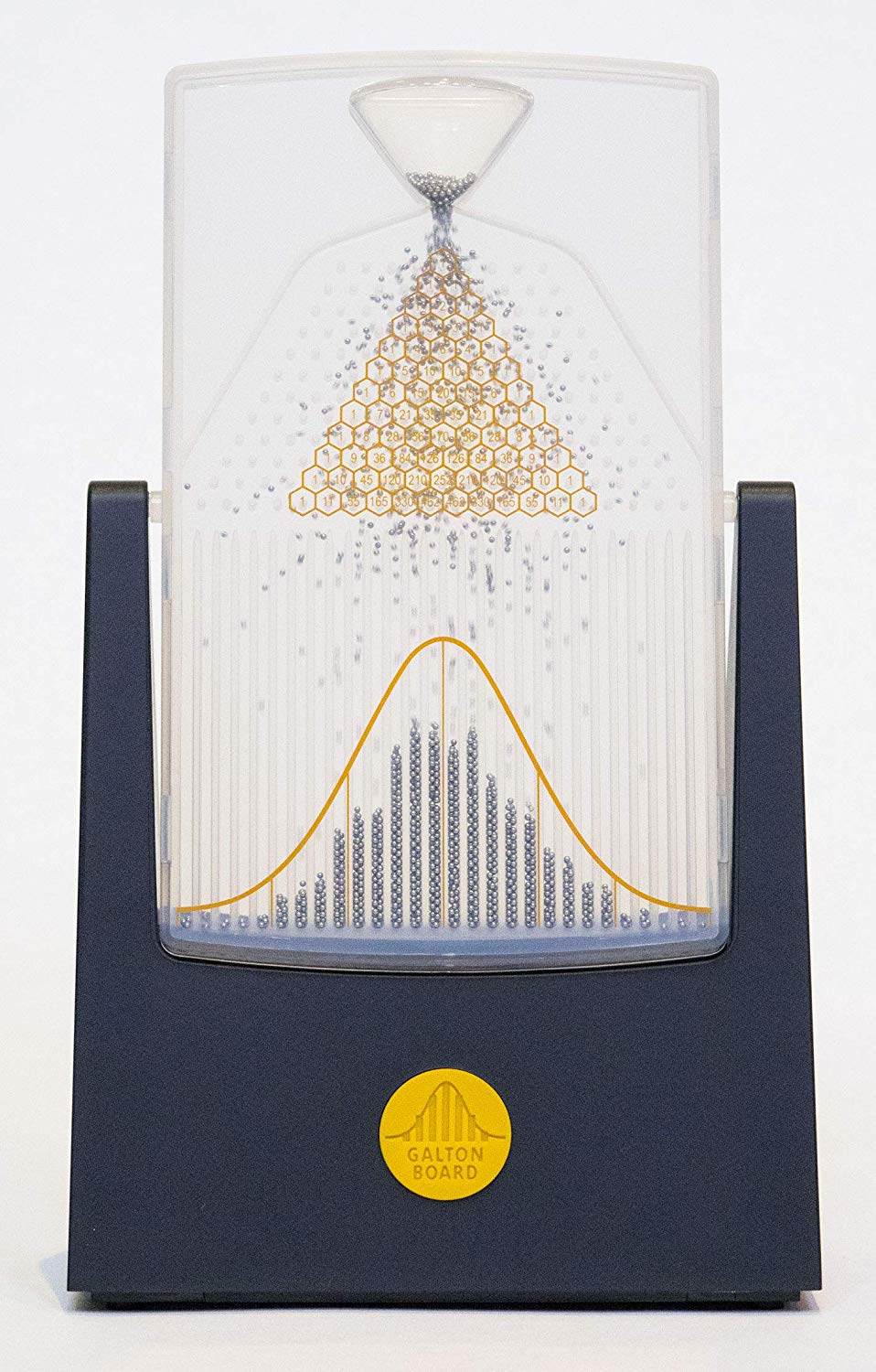
Get yours here, and be sure to check out Four Pines!
In Bayesian Spectacles' article, they discuss the Galton board and focus on model misspecification due to ignorance. They write
To elaborate, consider Jim, Johnny, and Jack who are standing side to side in front of a coin tossing machine. Their task is to predict the outcome of the next flip. Jim only knows the coin is fair, and he states the probability of the coin landing tails on the next flip is 50%. Johnny is taller than Jim and can just glimpse that the machine's container has the coin "tails up"; Johnny uses this knowledge to make a more precise prediction, namely that the requested probability is 51%. Jack, however, is even taller than Johnny, so can also see the machine's input panel that shows the set vertical force and angular momentum. This knowledge allows Jack to predict the outcome with much more certainty than Jim and Johnny. It seems to me that both Jim and Johnny's models are misspecified; had they known what Jack knows, they would have incorporated that information and adjusted their predictions accordingly. But even Jack's model is misspecified, in the sense that one may imagine a fourth person -James, say- who also knows the air resistance, which helps sharpen the prediction even more. This game of inventing hypothetical people who have access to additional informative predictors can continue until the chance of the next flip coming up tails is either 0 or 1. At that point, the model is no longer misspecified, but it has also stopped being statistical.
There are a couple of points to make here.
First, well-designed experiments over time, not beliefs, tend to reveal truth. A "likelihood swamping the prior" effect is why Jim's, Johnny's, Jack's, and James' beliefs due to their knowledge may not matter other than being interesting to them. If you get persons A, B, ... , Z, AA, BB, ... , ZZ, AAA, BBB, ..., ZZZ, etc., and graph their beliefs, they'd probably form something like a normal distribution. Moreover, having more information doesn't mean having quality information nor imply one is correct just because they have more information. The POTUS at this time has access to more information than you or I but that doesn't guarantee he is making the best decisions...for example.
Second, the jury is still out on the universe being literally deterministic or not. We might not even be able to ever specify everything involved in something as simple as a Galton board to get a 0 or 1 result, let alone more complex systems. Insert standard quantum mechanics handwaving arguments here, like not being able to determine the momentum and position of a particle at the same time. We may never be able to eliminate all types of uncertainties, which seems to be the case.
Third, even being able to say something is misspecified means that someone knows what the "true" situation is. In almost all interesting cases, we do not know that, hence the reason for statistics in the first place. In their article, they said that only Jim "knows" the coin is fair. How?
Last, a binomial or normal distribution being off from "truth" is quite OK and actually expected. There are the fields of robust and nonparametric statistics, as well as, of course, all other kinds of distributions available to use. One distribution being technically "wrong" for the job (something we'd only know if we knew an unknowable "truth") is no grounds for believing that another won't suffice or that the distribution itself is not useful in that situation. That is, Johnny having more information available does not mean that the normal distribution wouldn't be a great approximation to get the job done.
The Galton board demonstrates objectivity. Anyone, regardless of beliefs or Bayesian priors, can tip it over and see the results. Moreover, the sheer number of applications to different areas of life show frequentism working pretty well, and this despite any possible misspecification that we can't possibly know in most cases.
If Bayesian Spectacles (rose colored perhaps?) is free to invent hypothetical people (even though they are presumably against the hypothetical counterfactual data of frequentism even though no Bayesian actually observes all data in a prior distribution nor the thousands of MCMC realizations, but I digress!) then you should feel free to invent hypotheticals that prevent the hypothetical people from knowing all the information about the universe or any system.
Thanks for reading.
Please anonymously VOTE on the content you have just read:
Like:Dislike: